最大流量和线性分配问题
前言
这周收到的是个算法方面的,之前没接触过,算是当扩展视野了。
原文:Maximum Flow and the Linear Assignment Problem 作者: DMITRI IVANOVICH ARKHIPOV
文章正文
这里有一个问题:你的业务分配给承包商来履行合同。您可以浏览您的名单,并决定哪些承包商可以参与一个月的合同,你可以查看你的合同,看看哪些合同是一个月的任务。鉴于你知道每个承包商如何有效地履行每个合同,你如何分配承包商来最大化这个月的整体效益? 这是分配问题的一个例子,问题可以用古典的匈牙利算法( Hungarian algorithm)来解决。 匈牙利算法(也称为kuhn-munkres算法)是一种多项式时间算法,使加权二分图中的权重匹配最大化。在这里,承包商和合同可以被模型化为二分图,,它们的效力是承包商和合同节点之间的边权值。 在本文中,您将了解使用Edmonds-Karp算法解决线性分配问题的匈牙利算法的实现。您还将了解Edmonds-Karp算法如何对Ford-Fulkerson方法进行轻微修改,以及该修改如何重要。
最大流量问题
最大流量问题本身可以被非正式地描述为将流体或气体通过管道网络从单个源流到单个终端的问题。这样做的前提是,网络中的压力足以确保流体或气体不能在任何长度的管道或管道接头内逗留(这些管道的长度不同)。 通过对图进行某些更改,分配问题可以转化为最大的流问题。
准备工作
在许多数学和工程学科中出现了解决这些问题的想法,通常类似的概念被以不同的名称命名,并以不同的方式表达(例如,邻接矩阵和邻接列表)。由于这些概念是相当深奥的,所以一般根据给定的环境如何选择这些概念。 本文不会呈现任何超出引言中的集合理论的先验知识。 这篇文章中的实现代表了有向图(digraph)的问题。
有向图(DiGraphs)
有向图具有两个属性:setOfNodes 和setOfArcs。这两个属性都是集合(无序集合)。在这篇文章的代码块中,我实际上是使用Python的frozenset,但这个细节并不是特别重要。
DiGraph = namedtuple('DiGraph', ['setOfNodes','setOfArcs'])
(注意:本文中的所有代码都是用Python 3.6编写的。)
节点(Nodes)
一个节点n由两个属性组成:
n.uid
:唯一标识符。
这意味着对于任何两个节点x
和y
,
x.uid != y.uid
n.datum
:这表示任何数据对象。
Node = namedtuple('Node', ['uid','datum'])
弧(Arcs)
a.fromNode
:这是一个如上定义的节点。a.toNode
:这是一个如上定义的节点。a.datum
:这表示任何数据对象。
Arc = namedtuple('Arc', ['fromNode','toNode','datum'])
在digraph中的一组弧表示在digraph中的节点上的一个关二元关系。存在的弧a
意味着a.fromNode
和a.toNode
之间存在着一种关系。 在一个有向图(相对于无向图)中,存在与a.fromNode
和a.toNode
之间的关系不意味着类似的关系存在于a.toNode
和a.fromNode
之间。 这是因为在无向图中,所表达的关系不一定是对称的。
DiGraphs
节点和弧可用于定义有向图,但为方便起见,在下面的算法中,将使用字典(dictionary)来表示有向图。 下面是一种方法,可以将上面的graph表示转换成类似于此的字典表示:
def digraph_to_dict(G):
G_as_dict = dict([])
for a in G.setOfArcs:
if(a.fromNode not in G.setOfNodes):
err_msg = 'There is no Node {a.fromNode.uid!s} to match the Arc from {a.fromNode.uid!s} to {a.toNode.uid!s}'.format(**locals())
pdg(G)
raise KeyError(err_msg)
if(a.toNode not in G.setOfNodes):
err_msg = 'There is no Node {a.toNode.uid!s} to match the Arc from {a.fromNode.uid!s} to {a.toNode.uid!s}'.format(**locals())
pdg(G)
raise KeyError(err_msg)
G_as_dict[a.fromNode] = (G_as_dict[a.fromNode].union(frozenset([a]))) if (a.fromNode in G_as_dict) else frozenset([a])
for a in G.setOfArcs:
if(a.fromNode not in G.setOfNodes):
err_msg = 'There is no Node {a.fromNode.uid!s} to match the Arc from {a.fromNode.uid!s} to {a.toNode.uid!s}'.format(**locals())
raise KeyError(err_msg)
if a.toNode not in G_as_dict:
G_as_dict[a.toNode] = frozenset([])
return G_as_dict
2
3
4
5
6
7
8
9
10
11
12
13
14
15
16
17
18
19
这是另一个,把其转换成dictionaries中的dictionary,另一个有用的操作:
def digraph_to_double_dict(G):
G_as_dict = dict([])
for a in G.setOfArcs:
if(a.fromNode not in G.setOfNodes):
err_msg = 'There is no Node {a.fromNode.uid!s} to match the Arc from {a.fromNode.uid!s} to {a.toNode.uid!s}'.format(**locals())
raise KeyError(err_msg)
if(a.toNode not in G.setOfNodes):
err_msg = 'There is no Node {a.toNode.uid!s} to match the Arc from {a.fromNode.uid!s} to {a.toNode.uid!s}'.format(**locals())
raise KeyError(err_msg)
if(a.fromNode not in G_as_dict):
G_as_dict[a.fromNode] = dict({a.toNode : frozenset([a])})
else:
if(a.toNode not in G_as_dict[a.fromNode]):
G_as_dict[a.fromNode][a.toNode] = frozenset([a])
else:
G_as_dict[a.fromNode][a.toNode] = G_as_dict[a.fromNode][a.toNode].union(frozenset([a]))
for a in G.setOfArcs:
if(a.fromNode not in G.setOfNodes):
err_msg = 'There is no Node {a.fromNode.uid!s} to match the Arc from {a.fromNode.uid!s} to {a.toNode.uid!s}'.format(**locals())
raise KeyError(err_msg)
if a.toNode not in G_as_dict:
G_as_dict[a.toNode] = dict({})
return G_as_dict
2
3
4
5
6
7
8
9
10
11
12
13
14
15
16
17
18
19
20
21
22
23
24
当文章谈到由字典表示的有向图时,它将提及G_as_dict
来引用它。 有时,通过其 uid
(唯一标识符)从有向图G
获取节点是有帮助的:
def find_node_by_uid(find_uid, G):
nodes = {n for n in G.setOfNodes if n.uid == find_uid}
if(len(nodes) != 1):
err_msg = 'Node with uid {find_uid!s} is not unique.'.format(**locals())
raise KeyError(err_msg)
return nodes.pop()
2
3
4
5
6
在定义图时,人们有时会使用术语节点和顶点来引用相同的概念;对于弧和边来说也是一样的。 Python中两种流行的graph表示形式分别是使用字典和使用对象。本文中的表示介于这两种常用的表示之间。 是我的有向图表示。有很多类似的东西,但这个是我的。
Walks and Paths
S_Arcs
是一个在digraph G中的arcs的有限的序列(有序集合),这样假设a
是S_Arcs
中的除了最后一个外的任意一个弧,b
是 a
在这序列之后的,则在G.setOfNodes
中必然有一个 a.toNode = b.fromNode
这样的node n = a.fromNode
。 从S_Arcs
中的第一个arc 开始,直到S_Arcs
中的最后一个,收集(按照顺序)在s圆弧中每两个连续的弧之间定义的所有节点n。标记在此操作期间的收集的节点的有序集合 S_{Nodes}
。
def path_arcs_to_nodes(s_arcs):
s_nodes = list([])
arc_it = iter(s_arcs)
step_count = 0
last = None
try:
at_end = False
last = a1 = next(arc_it)
while (not at_end):
s_nodes += [a1.fromNode]
last = a2 = next(arc_it)
step_count += 1
if(a1.toNode != a2.fromNode):
err_msg = "Error at index {step_count!s} of Arc sequence.".format(**locals())
raise ValueError(err_msg)
a1 = a2
except StopIteration as e:
at_end = True
if(last is not None):
s_nodes += [last.toNode]
return s_nodes
2
3
4
5
6
7
8
9
10
11
12
13
14
15
16
17
18
19
20
21
22
23
- 如果在序列
S_Nodes
中任意一节点多次出现,S_Arcs
中一条在 digraphG
的Walk 。 - 反之,则称为
S_Arcs
中一条在digraphG
的从list(S_Nodes)[0]
到list(S_Nodes)[-1]
的 path 。
源点(Source Node)
称一个在digraph G
中的节点s
为源点,如果s
在 G.setOfNodes
,并且 G.setOfArcs
中不包含a.toNode = s
.的arc a
。
def source_nodes(G):
to_nodes = frozenset({a.toNode for a in G.setOfArcs})
sources = G.setOfNodes.difference(to_nodes)
return sources
2
3
4
终端点(Terminal Node)
称一个在digraph G
中的节点t
终端点,如果t
在 G.setOfNodes
,并且 G.setOfArcs
中不包含a.fromNode = t
.的arc a
。
def terminal_nodes(G):
from_nodes = frozenset({a.fromNode for a in G.setOfArcs})
terminals = G.setOfNodes.difference(from_nodes)
return terminals
2
3
4
Cuts and s-t Cuts
一个连通有向图 G
的cutcut
是来自G.setOfArcs
中arcs的一个子集。分割G
中一组节点nodes G.setOfNodes
。G
是连通的,如果每个node n
都在 G.setOfNodes
,并且至少有一个 arc a
在G.setOfArcs
,既不 n = a.fromNode
也不 n = a.toNode
,但 a.fromNode != a.toNode
。
Cut = namedtuple('Cut', ['setOfCutArcs'])
上面的定义指的是弧的一个子集,但是它也可以定义 G.setOfNodes
的节点的一个分区。 对于方法predecessors_of
和 successors_of
, n
是digraph G
的G.setOfNodes 中的一个节点,cut
is 是 G
的一个 cut :
def cut_predecessors_of(n, cut, G):
allowed_arcs = G.setOfArcs.difference(frozenset(cut.setOfCutArcs))
predecessors = frozenset({})
previous_count = len(predecessors)
reach_fringe = frozenset({n})
keep_going = True
while( keep_going ):
reachable_from = frozenset({a.fromNode for a in allowed_arcs if (a.toNode in reach_fringe)})
reach_fringe = reachable_from
predecessors = predecessors.union(reach_fringe)
current_count = len(predecessors)
keep_going = current_count - previous_count > 0
previous_count = current_count
return predecessors
def cut_successors_of(n, cut, G):
allowed_arcs = G.setOfArcs.difference(frozenset(cut.setOfCutArcs))
successors = frozenset({})
previous_count = len(successors)
reach_fringe = frozenset({n})
keep_going = True
while( keep_going ):
reachable_from = frozenset({a.toNode for a in allowed_arcs if (a.fromNode in reach_fringe)})
reach_fringe = reachable_from
successors = successors.union(reach_fringe)
current_count = len(successors)
keep_going = current_count - previous_count > 0
previous_count = current_count
return successors
2
3
4
5
6
7
8
9
10
11
12
13
14
15
16
17
18
19
20
21
22
23
24
25
26
27
28
29
参数cut
是 digraph G
的一个 cut :
def get_first_part(cut, G):
firstPartFringe = frozenset({a.fromNode for a in cut.setOfCutArcs})
firstPart = firstPartFringe
for n in firstPart:
preds = cut_predecessors_of(n,cut,G)
firstPart = firstPart.union(preds)
return firstPart
def get_second_part(cut, G):
secondPartFringe = frozenset({a.toNode for a in cut.setOfCutArcs})
secondPart = secondPartFringe
for n in secondPart:
succs= cut_successors_of(n,cut,G)
secondPart = secondPart.union(succs)
return secondPart
2
3
4
5
6
7
8
9
10
11
12
13
14
15
cut
是digraph G
的一个 cut 如果:
(get_first_part(cut, G).union(get_second_part(cut, G)) == G.setOfNodes) and (len(get_first_part(cut, G).intersect(get_second_part(cut, G))) == 0)
cut
是一个 x-y cut 如果:
(x in get_first_part(cut, G)) and (y in get_second_part(cut, G) ) and (x != y)
当一个节点 x
在一个 x-y cut cut
中是一个源点(source node),节点 y
在这个 x-y cut 中是一个终端点( terminal node), 则这个 cut 是一个 s-t cut。
STCut = namedtuple('STCut', ['s','t','cut'])
Flow Networks
你可以使用一个digraph G
表示一个flow Networks。 分配每个节点 n
,其中n
在G.setOfNodes
中,并且n.datum
是一个FlowNodeDatum
:
FlowNodeDatum = namedtuple('FlowNodeDatum', ['flowIn','flowOut'])
分配每个弧 a
, 其中 a
在 G.setOfArcs
中,并且 a.datum
是一个 FlowArcDatum
.:
FlowArcDatum = namedtuple('FlowArcDatum', ['capacity','flow'])
flowNodeDatum.flowIn
和flowNodeDatum.flowOut
是正 实数。 flowArcDatum.capacit
和flowArcDatum.flow
也正实数。 对于每一个在G.setOfNodes
中的节点 n
:
n.datum.flowIn = sum({a.datum.flow for a in G.Arcs if a.toNode == n})
n.datum.flowOut = sum({a.datum.flow for a in G.Arcs if a.fromNode == n})
2
Digraph G
现在代表一个flow network。 G
中的flow指在G
的所有弧 a
的a.flow
。
Feasible Flows
让 digraph G
代表一个 flow network。 被 G
代表的 flow network具有feasible flows,如果:
- 1、
G.setOfNodes
中除了源点和终端点的所有noden
:n.datum.flowIn = n.datum.flowOut
。 - 2、
G.setOfNodes
中的每一个 arca
:a.datum.capacity <= a.datum.flow
。
条件1称为守恒约束( conservation constraint)。 条件2称为容量约束(capacity constraint)。
Cut Capacity
一个由 digraph G
表示的一个 flow network的源点(source node )为s
且终端点( terminal node)为 t
的 **s-t cut stCut
**的 cut capacity:
def cut_capacity(stCut, G):
part_1 = get_first_part(stCut.cut,G)
part_2 = get_second_part(stCut.cut,G)
s_part = part_1 if stCut.s in part_1 else part_2
t_part = part_1 if stCut.t in part_1 else part_2
cut_capacity = sum({a.datum.capacity for a in stCut.cut.setOfCutArcs if ( (a.fromNode in s_part) and (a.toNode in t_part) )})
return cut_capacity
2
3
4
5
6
7
最小容量切割(Minimum Capacity Cut)
设stCut = stCut(s,t,cut)
是一个由 digraph G
表示的一个 flow network的 s-t cut。 如果没有其他的 s-t cut stCutCompetitor
在这个 flow network中,stCut
就是其最小容量切割(minimum capacity cut ):
cut_capacity(stCut, G) < cut_capacity(stCutCompetitor, G)
Stripping the Flows Away
我想这指的是取一个digraph G的结果,并从G.setOfNodes
的所有节点和 G.setOfArcs
.中所有的弧中分离出所有的流量数据:
def strip_flows(G):
new_nodes= frozenset( (Node(n.uid, FlowNodeDatum(0.0,0.0)) for n in G.setOfNodes) )
new_arcs = frozenset([])
for a in G.setOfArcs:
new_fromNode = Node(a.fromNode.uid, FlowNodeDatum(0.0,0.0))
new_toNode = Node(a.toNode.uid, FlowNodeDatum(0.0,0.0))
new_arc = Arc(new_fromNode, new_toNode, FlowArcDatum(a.datum.capacity, 0.0))
new_arcs = new_arcs.union(frozenset([new_arc]))
return DiGraph(new_nodes, new_arcs)
2
3
4
5
6
7
8
9
最大流量问题
一个 flow network 表示为一个 digraph G
, 一个源点s
在 G.setOfNodes
以及一个终端点 t
在 G.setOfNodes
中, G
可以表示一个 maximum flow problem ,如果:
(len(list(source_nodes(G))) == 1) and (next(iter(source_nodes(G))) == s) and (len(list(terminal_nodes(G))) == 1) and (next(iter(terminal_nodes(G))) == t)
标记此表示:
MaxFlowProblemState = namedtuple('MaxFlowProblemState', ['G','sourceNodeUid','terminalNodeUid','maxFlowProblemStateUid'])
sourceNodeUid = s.uid
, terminalNodeUid = t.uid
, 以及 maxFlowProblemStateUid
是问题实例的标识符。
最大流量解决方案
让maxFlowProblem
代表最大流问题。maxFlowProblem
的解决方案可以由表示为 flow network 的digraph H
.来表示。 Digraph H
是输入python maxFlowProblem
的最大流量问题的可行解决方案,若:
- 1、
strip_flows(maxFlowProblem.G) == strip_flows(H)
。 - 2、
H
是一个 flow network,具有feasible flows。
如果除了1和2: 没有其他的flow network 可以被 digraph K
表示,如 strip_flows(G) == strip_flows(K)
and find_node_by_uid(t.uid,G).flowIn < find_node_by_uid(t.uid,K).flowIn
。 那么H
也是一个maxFlowProblem
的最佳的解决方案。 换句话说,可行的最大流解决方案可以用有向图表示,其中: 1、有向图 G
与相应的最大流问题是相同的,不同之处是,任意节点和弧的n.datum.flowIn
,n.datum.flowOut
和a.datum.flow
可以是不同的。 2、代表具有可行流量(feasible flows)的流网络(flow network )。 并且,如果另外,它可以代表最佳最大流量解决方案:
- 所述
flowIn
的节点对应于所述终端节点中的最大流问题是尽可能的大(当条件1和2都还满意)。
如果图 H
表示可行的最大流解决方案:find_node_by_uid(s.uid,H).flowOut = find_node_by_uid(t.uid,H).flowIn
这是从最大流量,最小切割定理(下面讨论)得出的。非正式地,由于H
假设有可行的流,这意味着在跨越任何(其他)节点 (守约约束)时,流既不能被创建(源节点 除外s
)也不能被“销毁”(在终端节点 除外)。t
由于最大流问题仅包含单个源节点 s
和单个终端节点 t
,在“创造”的所有流动s
在必须被“破坏” t
或流网络并不具有可行流(所述保护约束将被违反)。 使图 H
表示可行的最大流解决方案 ; 上面的值称为第一流量值的H
。 让:
mfps=MaxFlowProblemState(H, maxFlowProblem.sourceNodeUid, maxFlowProblem.terminalNodeUid, maxFlowProblem.maxFlowProblemStateUid)
这意味着mfps
是maxFlowProblem的
一个后继状态,这只是意味着mfps
是像maxFlowProblem
一样,不同之处在于maxFlowProblem.setOfArcs
中弧a
的a.flow
的值与mfps.setOfArcs
中弧a
的 a.flow
的值不同。
def get_mfps_flow(mfps):
flow_from_s = find_node_by_uid(mfps.sourceNodeUid,mfps.G).datum.flowOut
flow_to_t = find_node_by_uid(mfps.terminalNodeUid,mfps.G).datum.flowIn
if( (flow_to_t - flow_from_s) > 0):
raise Exception('Infeasible s-t flow')
return flow_to_t
2
3
4
5
6
7
这是一个mfps
及其相关的maxFlowProb
.的可视化。图像中的每个弧 a
都有一个标签,这些标签是a.datum.flowFrom / a.datum.flowTo
,图像中的每个节点 n
都有一个标签,这些标签是n.uid {n.datum.flowIn / a.datum.flowOut}
。
s-t Cut Flow
让mfps
代表MaxFlowProblemState
,让stCut
代表cut的 mfps.G
。所述 cut flow的stCut
定义为:
def get_stcut_flow(stCut,mfps):
s = find_node_by_uid(mfps.sourceNodeUid, mfps.G)
t = find_node_by_uid(mfps.terminalNodeUid, mfps.G)
part_1 = get_first_part(stCut.cut,mfps.G)
part_2 = get_second_part(stCut.cut,mfps.G)
s_part = part_1 if s in part_1 else part_2
t_part = part_1 if t in part_1 else part_2
s_t_sum = sum(a.datum.flow for a in mfps.G.setOfArcs if (a in stCut.cut.setOfCutArcs) and (a.fromNode in s_part) )
t_s_sum = sum(a.datum.flow for a in mfps.G.setOfArcs if (a in stCut.cut.setOfCutArcs) and (a.fromNode in t_part) )
cut_flow = s_t_sum - t_s_sum
return cut_flow
2
3
4
5
6
7
8
9
10
11
st cut流是从包含源节点的分区到包含终端节点的分区的流的总和减去从包含终端节点的分区到包含源节点的分区的流的总和。
最大流量,最小切割
让maxFlowProblem
问题代表一个最大的流问题,让 maxFlowProblem
的解决方案用一个由表示为digraph H
的flow network来表示。 因为在最大流量问题流中只起源于单个源节点并终止于单个终端节点,并且由于容量限制和保护约束,我们知道进入的所有流maxFlowProblem.terminalNodeUid
必须经过任何s-t cut, 特别是它必须交叉minStCut
。意即:
get_flow_value(H, maxFlowProblem) <= cut_capacity(minStCut, maxFlowProblem.G)
解决最大流量问题
解决最大流问题的基本思想是,从一个由digraph H表示的最大流解决方案开始,这样的起始点可以是 H = strip_flows(maxFlowProblem.G)
。接下来的任务是使用 H
和通过对 H.setOfArcs
中 一些 arcs a
的 a.datum.flow
的值一些的贪婪修改,生成另一个由 digraph K
s表示的最大流量的解决方案,使得K不能仍然表示具有feasible flows and get_flow_value(H, maxFlowProblem) < get_flow_value(K, maxFlowProblem)
的 flow network。只要这个过程还在继续,那么最近遇到的最大流量的解决方案(K)的质量(get_flow_value(K, maxFlowProblem)
) )比找到的任何其他最大流量的解决方案都要好。如果进程到达一个点,它知道没有其他改进是可能的,那么这个过程就可以终止,它将返回最优的最大流量的解决方案。 上面的描述是常规的,并且跳过许多证据,例如这样的过程是否可能或可能需要多长时间,我将给出更多的细节和算法。
最大流量,最小切数定理(The Max Flow, Min Cut Theorem)
从《Flows in Networks by Ford and Fulkerson》 一书中,最大流量,最小定理(定理5.1)的定义是:
For any network, the maximal flow value from
s
tot
is equal to the minimum cut capacity of all cuts separatings
andt
. 对于任何网络,从s到t的最大流量的值等于所有的切割分离s和t中的最小割容量。
在这篇文章中使用该定义,它转化为: 一个由有向图 H
表示的流网络表示的 maxFlowProblem
的解决方案是最佳的,如果:
get_flow_value(H, maxFlowProblem) == cut_capacity(minStCut, maxFlowProblem.G)
The Ford-Fulkerson Method and the Edmonds-Karp Algorithm
CLRS定义了Ford-Fulkerson方法(第26.2节):
FORD-FULKERSON-METHOD(G, s, t):
initialize flow f to 0
while there exists an augmenting path p in the residual graph G_f augment flow f along
2
3
残差图(Residual Graph)
表示为有向图 G
的流网络的残差图可以表示为有向图 G_f
:
ResidualDatum = namedtuple('ResidualDatum', ['residualCapacity','action'])
def agg_n_to_u_cap(n,u,G_as_dict):
arcs_out = G_as_dict[n]
return sum([a.datum.capacity for a in arcs_out if( (a.fromNode == n) and (a.toNode == u) ) ])
def agg_n_to_u_flow(n,u,G_as_dict):
arcs_out = G_as_dict[n]
return sum([a.datum.flow for a in arcs_out if( (a.fromNode == n) and (a.toNode == u) ) ])
def get_residual_graph_of(G):
G_as_dict = digraph_to_dict(G)
residual_nodes = G.setOfNodes
residual_arcs = frozenset([])
for n in G_as_dict:
arcs_from = G_as_dict[n]
nodes_to = frozenset([find_node_by_uid(a.toNode.uid,G) for a in arcs_from])
for u in nodes_to:
n_to_u_cap_sum = agg_n_to_u_cap(n,u,G_as_dict)
n_to_u_flow_sum = agg_n_to_u_flow(n,u,G_as_dict)
if(n_to_u_cap_sum > n_to_u_flow_sum):
flow = round(n_to_u_cap_sum - n_to_u_flow_sum, TOL)
residual_arcs = residual_arcs.union( frozenset([Arc(n,u,datum=ResidualDatum(flow, 'push'))]) )
if(n_to_u_flow_sum > 0.0):
flow = round(n_to_u_flow_sum, TOL)
residual_arcs = residual_arcs.union( frozenset([Arc(u,n,datum=ResidualDatum(flow, 'pull'))]) )
return DiGraph(residual_nodes, residual_arcs)
2
3
4
5
6
7
8
9
10
11
12
13
14
15
16
17
18
19
20
21
22
23
24
25
26
27
28
29
agg_n_to_u_cap(n,u,G_as_dict)
返回 G.setOfArcs
的一个所有弧 a
是当a.fromNode = n
和a.toNode = u
时的子集时的 a.datum.capacity
的和。 agg_n_to_u_cap(n,u,G_as_dict)
返回 G.setOfArcs
的一个所有弧 a
是当a.fromNode = n
和a.toNode = u
时的子集时的 a.datum.flow
的和。 简而言之,残差图 G_f
表示可以在有向图G
上执行的某些动作。 由有向图G
表示的流网络中G.setOfNodes
的每对节点 n,u
可以在剩余图 G_f
中产生0,1或2个弧。 1、这对n,u
在G_f
中不产生任何弧中如果没有弧 a
在G.setOfArcs
是a.fromNode = n
和a.toNode = u
。 2、所述一对n,u
产生G_f.setOfArcs
中的弧 a
,在其中a
表示弧标记的一个 push flow****弧从n
到u
a = Arc(n,u,datum=ResidualNode(n_to_u_cap_sum - n_to_u_flow_sum))
如果n_to_u_cap_sum > n_to_u_flow_sum
。 3、所述一对n,u
产生G_f.setOfArcs
中的弧 a
,在其中a
表示弧标记的一个 pull flow 弧从n
到u
a = Arc(n,u,datum=ResidualNode(n_to_u_cap_sum - n_to_u_flow_sum))
如果n_to_u_flow_sum > 0.0
。 每个 push flow****弧在G_f.setOfArcs
表示总的添加的作用x <= n_to_u_cap_sum - n_to_u_flow_sum
流向弧中的所述G.setOfArcs
的子集,其中弧 a
是在该子集中,如果a.fromNode = n
和a.toNode = u
。 每 pull flow 弧在G_f.setOfArcs
代表共减去的动作x <= n_to_u_flow_sum
流向G.setOfArcs
的子集弧,其中的弧 a
是在G.setOfArcs的
子集,当a.fromNode = n
和a.toNode = u
。 执行个别推或拉从动作G_f
上适用的圆弧中G
可能会产生一个流网络没有可行流,因为容量的限制或保护的限制可能会在生成被违反流网络。 这是可视化每个弧a
表示的可视化中的最大流解决方案的前一个示例可视化的残差图a.residualCapacity。
增强路径
我们maxFlowProblem
是一个最大流问题,并让G_f = get_residual_graph_of(G)
是maxFlowProblem.G
的剩余图。 一条maxFlowProblem
的增广路径 augmentingPath
是任何路径从find_node_by_uid(maxFlowProblem.sourceNode,G_f)
到find_node_by_uid(maxFlowProblem.terminalNode,G_f)
。 事实证明,可增广路径 augmentingPath
可以应用到一个由有向图 H
表示的最大流量解决方案生成另一有向图 K
表示的最大流量解决方案,其中get_flow_value(H, maxFlowProblem) < get_flow_value(K, maxFlowProblem)
如果H
不是最佳。 就是这样:
def augment(augmentingPath, H):
augmentingPath = list(augmentingPath)
H_as_dict = digraph_to_dict(H)
new_nodes = frozenset({})
new_arcs = frozenset({})
visited_nodes = frozenset({})
visited_arcs = frozenset({})
bottleneck_residualCapacity = min( augmentingPath, key=lambda a: a.datum.residualCapacity ).datum.residualCapacity
for x in augmentingPath:
from_node_uid = x.fromNode.uid if x.datum.action == 'push' else x.toNode.uid
to_node_uid = x.toNode.uid if x.datum.action == 'push' else x.fromNode.uid
from_node = find_node_by_uid( from_node_uid, H )
to_node = find_node_by_uid( to_node_uid, H )
load = bottleneck_residualCapacity if x.datum.action == 'push' else -bottleneck_residualCapacity
for a in H_as_dict[from_node]:
if(a.toNode == to_node):
test_sum = a.datum.flow + load
new_flow = a.datum.flow
new_from_node_flow_out = a.fromNode.datum.flowOut
new_to_node_flow_in = a.toNode.datum.flowIn
new_from_look = {n for n in new_nodes if (n.uid == a.fromNode.uid)}
new_to_look = {n for n in new_nodes if (n.uid == a.toNode.uid) }
prev_from_node = new_from_look.pop() if (len(new_from_look)>0) else a.fromNode
prev_to_node = new_to_look.pop() if (len(new_to_look)>0) else a.toNode
new_nodes = new_nodes.difference(frozenset({prev_from_node}))
new_nodes = new_nodes.difference(frozenset({prev_to_node}))
if(test_sum > a.datum.capacity):
new_flow = a.datum.capacity
new_from_node_flow_out = prev_from_node.datum.flowOut - a.datum.flow + a.datum.capacity
new_to_node_flow_in = prev_to_node.datum.flowIn - a.datum.flow + a.datum.capacity
load = test_sum - a.datum.capacity
elif(test_sum < 0.0):
new_flow = 0.0
new_from_node_flow_out = prev_from_node.datum.flowOut - a.datum.flow
new_to_node_flow_in = prev_to_node.datum.flowIn - a.datum.flow
load = test_sum
else:
new_flow = test_sum
new_from_node_flow_out = prev_from_node.datum.flowOut - a.datum.flow + new_flow
new_to_node_flow_in = prev_to_node.datum.flowIn - a.datum.flow + new_flow
load = 0.0
new_from_node_flow_out = round(new_from_node_flow_out, TOL)
new_to_node_flow_in = round(new_to_node_flow_in, TOL)
new_flow = round(new_flow, TOL)
new_from_node = Node(prev_from_node.uid, FlowNodeDatum(prev_from_node.datum.flowIn, new_from_node_flow_out))
new_to_node = Node(prev_to_node.uid, FlowNodeDatum(new_to_node_flow_in, prev_to_node.datum.flowOut))
new_arc = Arc(new_from_node, new_to_node, FlowArcDatum(a.datum.capacity, new_flow))
visited_nodes = visited_nodes.union(frozenset({a.fromNode,a.toNode}))
visited_arcs = visited_arcs.union(frozenset({a}))
new_nodes = new_nodes.union(frozenset({new_from_node, new_to_node}))
new_arcs = new_arcs.union(frozenset({new_arc}))
not_visited_nodes = H.setOfNodes.difference(visited_nodes)
not_visited_arcs = H.setOfArcs.difference(visited_arcs)
full_new_nodes = new_nodes.union(not_visited_nodes)
full_new_arcs = new_arcs.union(not_visited_arcs)
G = DiGraph(full_new_nodes, full_new_arcs)
full_new_arcs_update = frozenset([])
for a in full_new_arcs:
new_from_node = a.fromNode
new_to_node = a.toNode
new_from_node = find_node_by_uid( a.fromNode.uid, G )
new_to_node = find_node_by_uid( a.toNode.uid, G )
full_new_arcs_update = full_new_arcs_update.union( {Arc(new_from_node, new_to_node, FlowArcDatum(a.datum.capacity, a.datum.flow))} )
G = DiGraph(full_new_nodes, full_new_arcs_update)
return G
2
3
4
5
6
7
8
9
10
11
12
13
14
15
16
17
18
19
20
21
22
23
24
25
26
27
28
29
30
31
32
33
34
35
36
37
38
39
40
41
42
43
44
45
46
47
48
49
50
51
52
53
54
55
56
57
58
59
60
61
62
63
64
65
66
67
68
69
70
71
72
73
74
75
76
77
在上面,TOL
是网络流量值舍入的一些公差值。这是为了避免级联不精确的浮点计算。所以,例如,我曾经TOL = 10
意味着一到十个有效数字。 让K = augment(augmentingPath, H)
,那么K
代表可行的最大流量的解决方案的maxFlowProblem
。为了使声明成真,所被K
表示的流网络必须具有可行的流(不违反容量约束或守恒约束)。 原因如下:在上面的方法中,每个节点添加到新的流网络表示为有向图 K
或者是完全相同的副本节点从有向图 H
或者一个节点 n
已经具有添加到其的相同数量n.datum.flowIn
作为它的n.datum.flowOut
。这意味着在K
中满足了保护约束条件就像在在H
中满足的。该 保护的约束是满足的,因为我们明确地检查,任何新的弧a
在网络中有a.datum.flow <= a.datum.capacity
; 因此,只要弧从集合H.setOfArcs
未经修改复制到K.setOfArcs
是不要违反容量约束,则K
不违反容量约束。 这也是真的,get_flow_value(H, maxFlowProblem) < get_flow_value(K, maxFlowProblem)
如果H
不是最佳的。 这是因为:对于增广路径 augmentingPath
中存在有向图中的表示剩余图 G_f
一的最大流问题 maxFlowProblem
,那么augmentingPath
上最后一个弧 a
必须是“推” 弧,它必须有a.toNode == maxFlowProblem.terminalNode
。一个增广路径被定义为一个终止于所述终端节点的最大流问题,这就是它的增广路径。从剩余图的定义可以看出,剩余图上的扩展路径中的最后一个弧必须是“推”弧因为任何“拉”的弧 b
的增广路径将b.toNode == maxFlowProblem.terminalNode
和b.fromNode != maxFlowProblem.terminalNode
来自定义路径。另外,从路径的定义来看,终端节点只能通过该augment
方法修改一次。因此,augment
修改maxFlowProblem.terminalNode.flowIn
一次,以及它增加的价值maxFlowProblem.terminalNode.flowIn
,因为最后弧中augmentingPath
必须是圆弧导致在修改maxFlowProblem.terminalNode.flowIn
过程中augment
。从定义augment
,因为它适用于“推” 弧时,maxFlowProblem.terminalNode.flowIn
只能增加,不能减少。
一些来自Sedgewick和Wayne的证明
《 Algorithms, fourth edition by Robert Sedgewich and Kevin Wayne 》一书提供了一些精彩和短暂的证据(第892-894页)。我将在这里重新创建它们,尽管我将使用以前定义的语言。对这些证明的标签与Sedgewick书中的相同。 命题E:对于任何有向图 H
表示一个可行的最大流量溶液的最大流问题 maxFlowProblem
,对于任何stCut
get_stcut_flow(stCut,H,maxFlowProblem) = get_flow_value(H, maxFlowProblem)
。 证明:让stCut=stCut(maxFlowProblem.sourceNode,maxFlowProblem.terminalNode,set([a for a in H.setOfArcs if a.toNode == maxFlowProblem.terminalNode]))
。命题E适用于stCut
从定义直接ST流量值。假设我们希望将一些节点 n
从s-partition(get_first_part(stCut.cut, G)
)移动到t分区中(get_second_part(stCut.cut, G))
,这样我们就需要改变stCut.cut
,这可能会改变stcut_flow = get_stcut_flow(stCut,H,maxFlowProblem)
和使命题E无效。但是,让我们看看stcut_flow
随着我们进行这种改变,价值的变化如何变化。节点 n
处于平衡,意味着流入节点 n
的总和等于其流出的总和(这对于H
表示可行的解决方案是必需的)。请注意,所有流量都是stcut_flow
进入节点 n
从s分区进入(t 分区的流进入节点 n
直接或间接地不会被计入该stcut_flow
值,因为它基于定义导向错误的方向)。此外,所有流出的流n
最终(直接或间接)流入终端节点(证明较早)。当我们将节点 移动n
到t分区中时,n
必须将从s分区进入的所有流都添加到新stcut_flow
值中; 但是,所有的流出都n
必须从新的中减去stcut_flow
值; 直接进入t分区的流标题的部分被减去,因为这个流现在是新的t分区的内部,不被计算为stcut_flow
。还必须从s分区中的从节点n
到节点的流的一部分被减去stcut_flow
:在n
移动到t分区之后,这些流将从t分区引导到s分区,因此不能占的stcut_flow
,因为这些流被去除流入的s分区,并且必须由这些流量的总和,并从S-分区流出到叔分区(其中来自ST所有流必须最终被减小)必须减少相同的金额。作为节点 n
在进程之前处于平衡状态,更新将在新stcut_flow
值减去时添加相同的值,从而使更新后的命题E为真。命题E的有效性随后从对t分区的大小的归纳来说。 以下是一些示例流网络,以帮助可视化命题E所持有的较不明显的情况; 在图像中,红色区域表示s分区,蓝色区域表示t分区,绿色弧表示st切割。在第二图像中,节点 A和节点 B 之间的流量增加,而进入终端节点 t 的流量不变: 推论:没有切割流量值可以超过任何切割的容量。 **命题F.(最大流量,最小定理):**让
f
一个 s-t flow。以下3个条件是等效的:
- 存在容量等于流量
f
的st切割。 f
是最大流量。- 没有增广路径相对
f
。
条件1意味着条件2由推论。条件2意味着条件3,因为增加路径的存在意味着具有较大值的流的存在,与最大化相矛盾f
。条件3意味着条件1:让C_s
是集合所有的节点,可以从到达s
与增广路径中剩余图。让C_t
剩下的弧,然后t
必须在C_t
(通过我们的假设)。所述弧从渡C_s
到C_t
随后形成ST切割仅包含弧 a
,其中任一a.datum.capacity = a.datum.flow
或a.datum.flow = 0.0
。如果这是另一种情况,则由一个弧连接的(由命题e)与C_s
的剩余剩余容量将在集合C_s
中,因为从s
到这样一个节点将会有一个增广路径。s-t cut的流量等于s-t cut的容量(因为从C_s
到C_t
的弧的流量等于容量),以及s-t流的值(由命题e) 这个关于最大流量,最小切割定理的表述,暗示了网络中流( Flows in Networks)的早期声明。 推论(完整性):当容量为整数时,存在一个整数最大流量,Ford-Fulkerson算法找到它。 证明:每个增加路径将流量增加一个正整数,“推” 弧中的未使用容量的最小值和“拉” 弧中的流量,所有这些都总是正整数。 这证明了来自CLRS的Ford-Fulkerson方法描述。该方法是继续寻找扩充路径并应用augment
于最新的maxFlowSolution
更好的解决方案,直到没有更多的增加路径意味着最新的最大流量解决方案是最佳的。
从Ford-Fulkerson 到 Edmonds-Karp
关于解决最大流量问题的其余问题有:
- 如何构建增强路径?
- 如果我们使用实数而不是整数,方法会终止吗?
- 要终止(如果有)需要多长时间?
所述Edmonds-Karp算法指定每个增强路径由构成广度优先搜索(BFS所述的)剩余图 ; 事实证明,上述第1点的决定也将迫使算法终止(点2),并允许确定渐近的时间和空间复杂性。 首先,这是一个BFS实现:
def bfs(sourceNode, terminalNode, G_f):
G_f_as_dict = digraph_to_dict(G_f)
parent_arcs = dict([])
visited = frozenset([])
deq = deque([sourceNode])
while len(deq) > 0:
curr = deq.popleft()
if curr == terminalNode:
break
for a in G_f_as_dict.get(curr):
if (a.toNode not in visited):
visited = visited.union(frozenset([a.toNode]))
parent_arcs[a.toNode] = a
deq.extend([a.toNode])
path = list([])
curr = terminalNode
while(curr != sourceNode):
if (curr not in parent_arcs):
err_msg = 'No augmenting path from {} to {}.'.format(sourceNode.uid, terminalNode.uid)
raise StopIteration(err_msg)
path.extend([parent_arcs[curr]])
curr = parent_arcs[curr].fromNode
path.reverse()
test = deque([path[0].fromNode])
for a in path:
if(test[-1] != a.fromNode):
err_msg = 'Bad path: {}'.format(path)
raise ValueError(err_msg)
test.extend([a.toNode])
return path
2
3
4
5
6
7
8
9
10
11
12
13
14
15
16
17
18
19
20
21
22
23
24
25
26
27
28
29
30
31
32
我使用了一个python集合模块中的deque。 为了回答上面的问题2,我将从Sedgewick和Wayne中提出另一个证明:命题G.在具有节点和弧的Edmonds-Karp算法中所需的增加路径数量最多。证明:每个扩充路径都有一个瓶颈弧 - 一个从残差图中删除的圆弧,因为它对应于一个“推” 弧,其被填充到容量或“拉” 弧,流动通过该弧逐渐变为0.每次弧变成瓶颈弧N
A
NA/2
中,任何长度增广路通过它必须由这是因为每个因子2增加节点在路径可能只出现一次或完全不(从定义路径)由于路径正在从最短探索路径到最长的意思是,至少有一个节点必须被通过特定瓶颈节点的下一个路径访问,这意味着在我们到达节点之前路径上的另外2个弧。由于扩充路径的长度至多为每个弧最多可以打开N``N/2
增广路径,和的总数增广路径是至多NA/2
。 该Edmonds-Karp算法执行O(NA^2)
。如果在大多数NA/2
的路径将在算法中进行探讨,探索每个路径与BFS是N+A
那么产品的最显著项,因此渐近复杂性O(NA^2)
。 我们mfp
是一个maxFlowProblemState
。
def edmonds_karp(mfp):
sid, tid = mfp.sourceNodeUid, mfp.terminalNodeUid
no_more_paths = False
loop_count = 0
while(not no_more_paths):
residual_digraph = get_residual_graph_of(mfp.G)
try:
asource = find_node_by_uid(mfp.sourceNodeUid, residual_digraph)
aterm = find_node_by_uid(mfp.terminalNodeUid, residual_digraph)
apath = bfs(asource, aterm, residual_digraph)
paint_mfp_path(mfp, loop_count, apath)
G = augment(apath, mfp.G)
s = find_node_by_uid(sid, G)
t = find_node_by_uid(tid, G)
mfp = MaxFlowProblemState(G, s.uid, t.uid, mfp.maxFlowProblemStateUid)
loop_count += 1
except StopIteration as e:
no_more_paths = True
return mfp
2
3
4
5
6
7
8
9
10
11
12
13
14
15
16
17
18
19
以上版本的效率并不高,O(NA^2)
因为它每次构建新的最大流解决方案和新的残差图(而不是随着算法进步而修改现有的有向图)。要得到真正的O(NA^2)
解决方案的算法必须保持两个有向图表示最大流问题的状态和其相关的剩余图。因此,该算法必须避免不必要地遍历弧和节点,并根据需要更新残差图中的值和相关值。 为了编写更快的Edmonds Karp算法,我从上面重写了几段代码。我希望通过生成新图的代码有助于了解发生了什么。在快速算法中,我使用了一些新的技巧和Python数据结构,我不想详细介绍。我会说,a.fromNode
和a.toNode
现在都被视为字符串和UID的,以节点。对于这段代码,让我们mfps
来一个maxFlowProblemState
import uuid
def fast_get_mfps_flow(mfps):
flow_from_s = {n for n in mfps.G.setOfNodes if n.uid == mfps.sourceNodeUid}.pop().datum.flowOut
flow_to_t = {n for n in mfps.G.setOfNodes if n.uid == mfps.terminalNodeUid}.pop().datum.flowIn
if( (flow_to_t - flow_from_s) > 0.00):
raise Exception('Infeasible s-t flow')
return flow_to_t
def fast_e_k_preprocess(G):
G = strip_flows(G)
get = dict({})
get['nodes'] = dict({})
get['node_to_node_capacity'] = dict({})
get['node_to_node_flow'] = dict({})
get['arcs'] = dict({})
get['residual_arcs'] = dict({})
for a in G.setOfArcs:
if(a.fromNode not in G.setOfNodes):
err_msg = 'There is no Node {a.fromNode.uid!s} to match the Arc from {a.fromNode.uid!s} to {a.toNode.uid!s}'.format(**locals())
raise KeyError(err_msg)
if(a.toNode not in G.setOfNodes):
err_msg = 'There is no Node {a.toNode.uid!s} to match the Arc from {a.fromNode.uid!s} to {a.toNode.uid!s}'.format(**locals())
raise KeyError(err_msg)
get['nodes'][a.fromNode.uid] = a.fromNode
get['nodes'][a.toNode.uid] = a.toNode
lark = Arc(a.fromNode.uid, a.toNode.uid, FlowArcDatumWithUid(a.datum.capacity, a.datum.flow, uuid.uuid4()))
if(a.fromNode.uid not in get['arcs']):
get['arcs'][a.fromNode.uid] = dict({a.toNode.uid : dict({lark.datum.uid : lark})})
else:
if(a.toNode.uid not in get['arcs'][a.fromNode.uid]):
get['arcs'][a.fromNode.uid][a.toNode.uid] = dict({lark.datum.uid : lark})
else:
get['arcs'][a.fromNode.uid][a.toNode.uid][lark.datum.uid] = lark
for a in G.setOfArcs:
if a.toNode.uid not in get['arcs']:
get['arcs'][a.toNode.uid] = dict({})
for n in get['nodes']:
get['residual_arcs'][n] = dict()
get['node_to_node_capacity'][n] = dict()
get['node_to_node_flow'][n] = dict()
for u in get['nodes']:
n_to_u_cap_sum = sum(a.datum.capacity for a in G.setOfArcs if (a.fromNode.uid == n) and (a.toNode.uid == u) )
n_to_u_flow_sum = sum(a.datum.flow for a in G.setOfArcs if (a.fromNode.uid == n) and (a.toNode.uid == u) )
if(n_to_u_cap_sum > n_to_u_flow_sum):
flow = round(n_to_u_cap_sum - n_to_u_flow_sum, TOL)
get['residual_arcs'][n][u] = Arc(n,u,ResidualDatum(flow, 'push'))
if(n_to_u_flow_sum > 0.0):
flow = round(n_to_u_flow_sum, TOL)
get['residual_arcs'][u][n] = Arc(u,n,ResidualDatum(flow, 'pull'))
get['node_to_node_capacity'][n][u] = n_to_u_cap_sum
get['node_to_node_flow'][n][u] = n_to_u_flow_sum
return get
def fast_bfs(sid, tid, get):
parent_of = dict([])
visited = frozenset([])
deq = coll.deque([sid])
while len(deq) > 0:
n = deq.popleft()
if n == tid:
break
for u in get['residual_arcs'][n]:
if (u not in visited):
visited = visited.union(frozenset({u}))
parent_of[u] = get['residual_arcs'][n][u]
deq.extend([u])
path = list([])
curr = tid
while(curr != sid):
if (curr not in parent_of):
err_msg = 'No augmenting path from {} to {}.'.format(sid, curr)
raise StopIteration(err_msg)
path.extend([parent_of[curr]])
curr = parent_of[curr].fromNode
path.reverse()
return path
def fast_edmonds_karp(mfps):
sid, tid = mfps.sourceNodeUid, mfps.terminalNodeUid
get = fast_e_k_preprocess(mfps.G)
no_more_paths, loop_count = False, 0
while(not no_more_paths):
try:
apath = fast_bfs(sid, tid, get)
get = fast_augment(apath, get)
loop_count += 1
except StopIteration as e:
no_more_paths = True
nodes = frozenset(get['nodes'].values())
arcs = frozenset({})
for from_node in get['arcs']:
for to_node in get['arcs'][from_node]:
for arc in get['arcs'][from_node][to_node]:
arcs |= frozenset({get['arcs'][from_node][to_node][arc]})
G = DiGraph(nodes, arcs)
mfps = MaxFlowProblemState(G, sourceNodeUid=sid, terminalNodeUid=tid, maxFlowProblemStateUid=mfps.maxFlowProblemStateUid)
return mfps
2
3
4
5
6
7
8
9
10
11
12
13
14
15
16
17
18
19
20
21
22
23
24
25
26
27
28
29
30
31
32
33
34
35
36
37
38
39
40
41
42
43
44
45
46
47
48
49
50
51
52
53
54
55
56
57
58
59
60
61
62
63
64
65
66
67
68
69
70
71
72
73
74
75
76
77
78
79
80
81
82
83
84
85
86
87
88
89
90
91
92
93
94
95
96
97
98
99
100
101
102
103
104
105
106
这是一个可视化的方法,该算法如何从上面解决示例流网络。可视化示出,因为它们都反映在步骤有向图 G
表示的最先进的最新流网络并且当它们被反映在剩余图那个流的网络。增广路径中剩余图被示出为红色的路径,并且有向图表示的问题的一组节点和弧的影响由给定的增广路径以绿色突出显示。在每种情况下,我将突出显示将要更改的图形部分(红色或绿色),然后在更改后显示图形(黑色)。 这是另一种可视化的算法,解决了不同的示例流网络。请注意,这一个使用实数,并包含多个具有相同
fromNode
和toNode
的值的弧。 **还要注意,由于具有“拉”的残差可能是扩展路径的一部分,所以在流网络的DiGraph中受影响的节点可能不在路径中G!
。
双边图
假设我们有一个有向图 G
,G
是二部是否有可能进行分区节点中G.setOfNodes
分成两组(part_1
和part_2
),使得对于任何电弧 a
在G.setOfArcs
它不可能是真的是a.fromNode
在part_1
和a.toNode
中part_1
。它也不可能是真的是a.fromNode
在part_2
和a.toNode
中part_2
。 换句话说G
是二分,如果它可以被划分成两个集合的节点,使得每个弧必须连接一个节点中的一组的节点中的其他组。
测试双边
假设我们有一个图 G
,我们想测试它是否是二分的。我们可以O(|G.setOfNodes|+|G.setOfArcs|)
通过贪婪地将图形着色为两种颜色来做到这一点。 首先,我们需要生成一个新的有向图 H
。该图将有将有相同的一组节点的G
,但将有更多的弧比G
。G
的每一个弧 a
将在H
中创建2个弧; 第一个弧将相同a
,第二个弧反转a
(b = Arc(a.toNode,a.fromNode,a.datum)
)的导向器。
Bipartition = coll.namedtuple('Bipartition',['firstPart', 'secondPart', 'G'])
def bipartition(G):
nodes = frozenset(G.setOfNodes
arcs = frozenset(G.setOfArcs)
arcs = arcs.union( frozenset( {Arc(a.toNode,a.fromNode,a.datum) for a in G.setOfArcs} ) )
H = DiGraph(nodes, arcs)
H_as_dict = digraph_to_dict(H)
color = dict([])
some_node = next(iter(H.setOfNodes))
deq = coll.deque([some_node])
color[some_node] = -1
while len(deq) > 0:
curr = deq.popleft()
for a in H_as_dict.get(curr):
if (a.toNode not in color):
color[a.toNode] = -1*color[curr]
deq.extend([a.toNode])
elif(color[curr] == color[a.toNode]):
print(curr,a.toNode)
raise Exception('Not Bipartite.')
firstPart = frozenset( {n for n in color if color[n] == -1 } )
secondPart = frozenset( {n for n in color if color[n] == 1 } )
if( firstPart.union(secondPart) != G.setOfNodes ):
raise Exception('Not Bipartite.')
return Bipartition(firstPart, secondPart, G)
2
3
4
5
6
7
8
9
10
11
12
13
14
15
16
17
18
19
20
21
22
23
24
25
26
27
28
最大双方匹配
甲最大二分匹配是一个最大匹配上的有向图 G
,其是二分。 鉴于G
是二分,寻找的问题最大双边匹配可以转化为一个最大流问题可解与埃德蒙斯-卡普算法,然后将最大双边匹配可以从溶液到回收最大流问题。 我们bipartition
是一个bipartition的G
。 为了做到这一点,我需要生成一个新的图(H
)和一些新的节点(H.setOfNodes
)和一些新的弧(H.setOfArcs
)。H.setOfNodes
包含的所有节点中G.setOfNodes
,另外两个nodess,s
(一个源节点)和t
(一个终端节点)。 H.setOfArcs
将为每个包含一个弧G.setOfArcs
。如果一个弧线 a
处于G.setOfArcs
并且a.fromNode
处于bipartition.firstPart
并且a.toNode
在其中bipartition.secondPart
并且包括a
在H
(添加FlowArcDatum(1,0)
)中)。 如果a.fromNode
是在bipartition.secondPart
和a.toNode
在bipartition.firstPart
,然后包括Arc(a.toNode,a.fromNode,FlowArcDatum(1,0))
在H.setOfArcs
。 二分图的定义确保没有弧连接两个节点位于同一分区中的任何节点。还包含一个弧从节点到每个节点在。最后,包含一个弧的每个节点中,以节点。为所有在。H.setOfArcs
s``bipartition.firstPart``H.setOfArcs``bipartition.secondPart
t``a.datum.capacity = 1``a``H.setOfArcs
第一分区中的节点在G.setOfNodes
两个不相交的集合(part1
和part2
),使得没有电弧在G.setOfArcs
从一组涉及相同组(在此分区是可能的,因为G
是二分)。接着,新增弧在G.setOfArcs
其从直接part1
到part2
成H.setOfArcs
。然后创建单个源节点 s
和单个终端节点, t
并创建一些更多的弧 然后,构建一个maxFlowProblemState
。
def solve_mbm( bipartition ):
s = Node(uuid.uuid4(), FlowNodeDatum(0,0))
t = Node(uuid.uuid4(), FlowNodeDatum(0,0))
translate = {}
arcs = frozenset([])
for a in bipartition.G.setOfArcs:
if ( (a.fromNode in bipartition.firstPart) and (a.toNode in bipartition.secondPart) ):
fark = Arc(a.fromNode,a.toNode,FlowArcDatum(1,0))
arcs = arcs.union({fark})
translate[frozenset({a.fromNode.uid,a.toNode.uid})] = a
elif ( (a.toNode in bipartition.firstPart) and (a.fromNode in bipartition.secondPart) ):
bark = Arc(a.toNode,a.fromNode,FlowArcDatum(1,0))
arcs = arcs.union({bark})
translate[frozenset({a.fromNode.uid,a.toNode.uid})] = a
arcs1 = frozenset( {Arc(s,n,FlowArcDatum(1,0)) for n in bipartition.firstPart } )
arcs2 = frozenset( {Arc(n,t,FlowArcDatum(1,0)) for n in bipartition.secondPart } )
arcs = arcs.union(arcs1.union(arcs2))
nodes = frozenset( {Node(n.uid,FlowNodeDatum(0,0)) for n in bipartition.G.setOfNodes} ).union({s}).union({t})
G = DiGraph(nodes, arcs)
mfp = MaxFlowProblemState(G, s.uid, t.uid, 'bipartite')
result = edmonds_karp(mfp)
lookup_set = {a for a in result.G.setOfArcs if (a.datum.flow > 0) and (a.fromNode.uid != s.uid) and (a.toNode.uid != t.uid)}
matching = {translate[frozenset({a.fromNode.uid,a.toNode.uid})] for a in lookup_set}
return matching
2
3
4
5
6
7
8
9
10
11
12
13
14
15
16
17
18
19
20
21
22
23
24
25
26
27
28
最小节点覆盖
有向图 G
中的节点覆盖是一组节点(cover
),G.setOfNodes
从而使得对于任何一个弧 a
都G.setOfArcs
必须为true (a.fromNode in cover) or (a.toNode in cover)
。 最小节点覆盖是图中仍然是节点覆盖的最小可能的节点集合。König定理表明,在二分图中,该图上最大匹配的大小等于最小节点覆盖的大小,并且它表明节点覆盖可以如何从最大匹配恢复: 假设我们有二分区 bipartition
和最大匹配 matching
。定义一个新的有向图 H
,H.setOfNodes=G.setOfNodes
中,圆弧的H.setOfArcs
是两个集合的并集。 第一组是弧 a
中matching
,与变化,如果a.fromNode in bipartition.firstPart
和a.toNode in bipartition.secondPart
然后a.fromNode
和a.toNode
在所生成的换弧给出这样的弧一个a.datum.inMatching=True
属性,以指示它们是从衍生弧在一个匹配。 第二组是弧 a
NOT中matching
,用的是,如果改变a.fromNode in bipartition.secondPart
和a.toNode in bipartition.firstPart
然后a.fromNode
与a.toNode
被在所创建的交换弧(给出这样弧一个a.datum.inMatching=False
属性)。 接下来,运行一个深度优先搜索(DFS)从各起始节点 n
中bipartition.firstPart
既不是n == a.fromNode
也不n == a.toNodes
进行任何弧 a
中matching
。在DFS期间,某些节点被访问,有些节点不存在(将该信息存储在一个n.datum.visited
字段中)。该最小顶点覆盖是联盟节点 {a.fromNode for a in H.setOfArcs if ( (a.datum.inMatching) and (a.fromNode.datum.visited) ) }
和节点 {a.fromNode for a in H.setOfArcs if (a.datum.inMatching) and (not a.toNode.datum.visited)}
。 这可以证明从导致最大匹配到一个最小的顶点覆盖由反证法,需要一定的弧度 a
是假想不是盖的,并考虑是否就四个案件都a.fromNode
和a.toNode
属于(无论是作为toNode
或fromNode
)任何电弧的匹配 matching
。每个案例导致矛盾归因于DFS访问的顺序节点,而且事实上matching
是一个最大匹配。 假设我们有一个函数来执行这些步骤,并返回包含最小节点覆盖的节点集,当给定有向图G
时,**最大匹配 **matching
:
ArcMatchingDatum = coll.namedtuple('ArcMatchingDatum', ['inMatching' ])
NodeMatchingDatum = coll.namedtuple('NodeMatchingDatum', ['visited'])
def dfs_helper(snodes, G):
sniter, do_stop = iter(snodes), False
visited, visited_uids = set(), set()
while(not do_stop):
try:
stack = [ next(sniter) ]
while len(stack) > 0:
curr = stack.pop()
if curr.uid not in visited_uids:
visited = visited.union( frozenset( {Node(curr.uid, NodeMatchingDatum(False))} ) )
visited_uids = visited.union(frozenset({curr.uid}))
succ = frozenset({a.toNode for a in G.setOfArcs if a.fromNode == curr})
stack.extend( succ.difference( frozenset(visited) ) )
except StopIteration as e:
stack, do_stop = [], True
return visited
def get_min_node_cover(matching, bipartition):
nodes = frozenset( { Node(n.uid, NodeMatchingDatum(False)) for n in bipartition.G.setOfNodes} )
G = DiGraph(nodes, None)
charcs = frozenset( {a for a in matching if ( (a.fromNode in bipartition.firstPart) and (a.toNode in bipartition.secondPart) )} )
arcs0 = frozenset( { Arc(find_node_by_uid(a.toNode.uid,G), find_node_by_uid(a.fromNode.uid,G), ArcMatchingDatum(True) ) for a in charcs } )
arcs1 = frozenset( { Arc(find_node_by_uid(a.fromNode.uid,G), find_node_by_uid(a.toNode.uid,G), ArcMatchingDatum(True) ) for a in matching.difference(charcs) } )
not_matching = bipartition.G.setOfArcs.difference( matching )
charcs = frozenset( {a for a in not_matching if ( (a.fromNode in bipartition.secondPart) and (a.toNode in bipartition.firstPart) )} )
arcs2 = frozenset( { Arc(find_node_by_uid(a.toNode.uid,G), find_node_by_uid(a.fromNode.uid,G), ArcMatchingDatum(False) ) for a in charcs } )
arcs3 = frozenset( { Arc(find_node_by_uid(a.fromNode.uid,G), find_node_by_uid(a.toNode.uid,G), ArcMatchingDatum(False) ) for a in not_matching.difference(charcs) } )
arcs = arcs0.union(arcs1.union(arcs2.union(arcs3)))
G = DiGraph(nodes, arcs)
bip = Bipartition({find_node_by_uid(n.uid,G) for n in bipartition.firstPart},{find_node_by_uid(n.uid,G) for n in bipartition.secondPart},G)
match_from_nodes = frozenset({find_node_by_uid(a.fromNode.uid,G) for a in matching})
match_to_nodes = frozenset({find_node_by_uid(a.toNode.uid,G) for a in matching})
snodes = bip.firstPart.difference(match_from_nodes).difference(match_to_nodes)
visited_nodes = dfs_helper(snodes, bip.G)
not_visited_nodes = bip.G.setOfNodes.difference(visited_nodes)
H = DiGraph(visited_nodes.union(not_visited_nodes), arcs)
cover1 = frozenset( {a.fromNode for a in H.setOfArcs if ( (a.datum.inMatching) and (a.fromNode.datum.visited) ) } )
cover2 = frozenset( {a.fromNode for a in H.setOfArcs if ( (a.datum.inMatching) and (not a.toNode.datum.visited) ) } )
min_cover_nodes = cover1.union(cover2)
true_min_cover_nodes = frozenset({find_node_by_uid(n.uid, bipartition.G) for n in min_cover_nodes})
return min_cover_nodes
2
3
4
5
6
7
8
9
10
11
12
13
14
15
16
17
18
19
20
21
22
23
24
25
26
27
28
29
30
31
32
33
34
35
36
37
38
39
40
41
42
43
44
45
46
47
线性分配问题
线性分配问题包括在加权二分图中找到最大权重匹配。 像这个帖子一开始的问题可以表达为线性分配问题。给定一组工作人员,一组任务,以及一个指定一个工作人员分配到一个任务中的获利能力的功能,我们希望最大化所有作业的总和; 这是一个线性分配问题。 假设任务和工作人员的数量是相等的,虽然我会表明这个假设很容易被删除。在实现中,我代表弧的权重与属性a.datum.weight
为弧 a
。
WeightArcDatum = namedtuple('WeightArcDatum', [weight])
Kuhn-Munkres算法
Kuhn-Munkres算法解决了线性分配问题。一个很好的实现可能需要O(N^{4})
时间(代表问题的图中N
的节点数量)。更容易解释的实现(对于重新生成DiGraph的版本)和(用于维护DiGraph的版本)。这与Edmonds-Karp算法的两种不同实现类似。O(N^{5})``O(N^{4})
对于这个描述,我只使用完整的二分图(那些半分节点在二分区的一部分,另一半在第二部分)。在工作中,任务动机意味着工作人员和任务一样多。 这似乎是一个重要的条件(如果这些集合不平等的话),但很容易解决这个问题; 我在最后一节谈到如何做到这一点。 这里描述的算法的版本使用零重量弧的有用概念。不幸的是,当我们解决最小化(如果不是最大化我们的工作人员的任务任务,而不是最小化这样的任务的成本)时,这个概念才有意义。 幸运的是,通过将每个弧权重设置到哪里,很容易将最大线性分配问题转化为最小线性分配问题。原始最大化问题的解决方案将与弧权重更改后的解最小化问题相同。所以剩下的,假设我们做这个改变。 a``M-a.datum.weight``M=max({a.datum.weight for a in G.setOfArcs})
的库恩-的Munkres算法解决了最小重量以加权二分图匹配由序列最大匹配数中的未加权二分图。如果我们找到一个完美的匹配上有向图中的代表性线性分配问题,如果每个的重量弧的匹配是零,那么我们已经找到了最小重量的匹配,因为这种匹配表明,所有节点的有向图已经匹配由圆弧 具有最低可能的成本(根据先前的定义,无成本可低于0)。 没有其他弧可以添加到匹配(因为所有节点已经匹配),并且不应该从匹配中删除弧,因为任何可能的替换弧将至少具有相同的重量值。 如果我们发现其子图的最大匹配仅包含零重量弧,并且它不是完美匹配,则我们没有完整的解决方案(因为匹配不完美)。然而,我们可以产生一个新的有向图通过改变的权重的弧在该新0重量的方式弧出现和的最优解是相同的最优解。由于我们保证在每次迭代中产生至少一个零重量弧,所以我们保证我们将达到一个完美匹配G
H``G.setOfArcs``H``G
不超过**| G.setOfNodes | ^ {2} = N ^ {2}这样的迭代。 假设在二分法中** bipartition
, bipartition.firstPart
包含表示工作者的节点,并且bipartition.secondPart
表示代表任务的节点。 该算法首先生成一个新的有向图 H
。H.setOfNodes = G.setOfNodes
。一些弧线在H
从产生的节点 n
中bipartition.firstPart
。每一个这样的节点 n
产生一个电弧 b
在H.setOfArcs
每个弧 a
在bipartition.G.setOfArcs
其中a.fromNode = n
或a.toNode = n
,b=Arc(a.fromNode, a.toNode, a.datum.weight - z)
其中z=min(x.datum.weight for x in G.setOfArcs if ( (x.fromNode == n) or (x.toNode == n) ))
。 更电弧在H
从生成的节点 n
中bipartition.secondPart
。每一个这样的节点 n
产生一个电弧 b
在H.setOfArcs
每个弧 a
在bipartition.G.setOfArcs
其中a.fromNode = n
或a.toNode = n
,b=Arc(a.fromNode, a.toNode, ArcWeightDatum(a.datum.weight - z))
其中z=min(x.datum.weight for x in G.setOfArcs if ( (x.fromNode == n) or (x.toNode == n) ))
。 KMA: 接着,形成一个新的有向图 K
仅构成零个重量弧从H
和节点 入射在这些弧。形成bipartition
了对节点中K
,然后用solve_mbm( bipartition )
获得的最大匹配(matching
上)K
。如果matching
是一个完美匹配中H
(该圆弧在matching
顷入射所有上的节点中H.setOfNodes
),则matching
是到的最优解的线性分配问题。 否则,如果matching
是不完美的,产生最少的顶点覆盖的K
使用node_cover = get_min_node_cover(matching, bipartition(K))
。接下来,定义z=min({a.datum.weight for a in H.setOfArcs if a not in node_cover})
。定义nodes = H.setOfNodes
,arcs1 = {Arc(a.fromNode,a.toNode,ArcWeightDatum(a.datum.weigh-z)) for a in H.setOfArcs if ( (a.fromNode not in node_cover) and (a.toNode not in node_cover)}
,arcs2 = {Arc(a.fromNode,a.toNode,ArcWeightDatum(a.datum.weigh)) for a in H.setOfArcs if ( (a.fromNode not in node_cover) != (a.toNode not in node_cover)}
,arcs3 = {Arc(a.fromNode,a.toNode,ArcWeightDatum(a.datum.weigh+z)) for a in H.setOfArcs if ( (a.fromNode in node_cover) and (a.toNode in node_cover)}
。!=
上一个表达式中的符号用作XOR运算符。然后arcs = arcs1.union(arcs2.union(arcs3))
。接下来,H=DiGraph(nodes,arcs)
。回到标签KMA。该算法继续,直到产生完美的匹配。这种匹配也是线性分配问题的解决方案。
def kuhn_munkres( bipartition ):
nodes = bipartition.G.setOfNodes
arcs = frozenset({})
for n in bipartition.firstPart:
z = min( {x.datum.weight for x in bipartition.G.setOfArcs if ( (x.fromNode == n) or (x.toNode == n) )} )
arcs = arcs.union( frozenset({Arc(a.fromNode, a.toNode, ArcWeightDatum(a.datum.weight - z)) }) )
for n in bipartition.secondPart:
z = min( {x.datum.weight for x in bipartition.G.setOfArcs if ( (x.fromNode == n) or (x.toNode == n) )} )
arcs = arcs.union( frozenset({Arc(a.fromNode, a.toNode, ArcWeightDatum(a.datum.weight - z)) }) )
H = DiGraph(nodes, arcs)
assignment, value = dict({}), 0
not_done = True
while( not_done ):
zwarcs = frozenset( {a for a in H.setOfArcs if a.datum.weight == 0} )
znodes = frozenset( {n.fromNode for n in zwarcs} ).union( frozenset( {n.toNode for n in zwarcs} ) )
K = DiGraph(znodes, zwarcs)
k_bipartition = bipartition(K)
matching = solve_mbm( k_bipartition )
mnodes = frozenset({a.fromNode for a in matching}).union(frozenset({a.toNode for a in matching}))
if( len(mnodes) == len(H.setOfNodes) ):
for a in matching:
assignment[ a.fromNode.uid ] = a.toNode.uid
value = sum({a.datum.weight for a in matching})
not_done = False
else:
node_cover = get_min_node_cover(matching, bipartition(K))
z = min( frozenset( {a.datum.weight for a in H.setOfArcs if a not in node_cover} ) )
nodes = H.setOfNodes
arcs1 = frozenset( {Arc(a.fromNode,a.toNode,ArcWeightDatum(a.datum.weigh-z)) for a in H.setOfArcs if ( (a.fromNode not in node_cover) and (a.toNode not in node_cover)} )
arcs2 = frozenset( {Arc(a.fromNode,a.toNode,ArcWeightDatum(a.datum.weigh)) for a in H.setOfArcs if ( (a.fromNode not in node_cover) != (a.toNode not in node_cover)} )
arcs3 = frozenset( {Arc(a.fromNode,a.toNode,ArcWeightDatum(a.datum.weigh+z)) for a in H.setOfArcs if ( (a.fromNode in node_cover) and (a.toNode in node_cover)} )
arcs = arcs1.union(arcs2.union(arcs3))
H = DiGraph(nodes,arcs)
return value, assignment
2
3
4
5
6
7
8
9
10
11
12
13
14
15
16
17
18
19
20
21
22
23
24
25
26
27
28
29
30
31
32
33
34
35
36
37
38
39
40
这个实现是O(N^{5})
因为它在每个迭代中生成新的最大匹配 matching
; 类似于Edmonds-Karp的前两个实现,该算法可以被修改,使得它跟踪匹配并且智能地适应于每次迭代。当这样做时,复杂性就变成了O(N^{4})
。可以运行更高级和更新版本的该算法(需要一些更高级的数据结构)O(N^{3})
。在这篇文章中可以找到上述更简单的实现和更高级的实现的细节,这激发了这个博文。 对弧权重的任何操作都不会修改算法返回的最终分配。这是因为:由于我们的输入图形总是完全二部图的解决方案必须在每个映射节点在一个分区到另一个节点的第二个分区,通过电弧这两者之间的节点。请注意,在执行的操作圆弧权从未改变的顺序(按重量计订购)弧事件发表任何特定的节点。 因此,当算法以完美的完全二分匹配终止时,每个节点被分配零权重弧,因为在算法期间来自该节点的弧的相对顺序没有改变,并且由于零权重弧是最便宜的可能的弧,在完美完成二分匹配保证了一个这样的弧线存在于每个节点。这意味着生成的解决方案与原始的线性分配问题的解决方案确实是一样的,没有任何修改弧权重。
不平衡分配
似乎算法是非常有限的,因为它只描述在完整的二分图上(其中一半的节点在二分区的一部分中,另一半在第二部分中)。在工作中,任务动机意味着工作人员与任务一样多(似乎很有限制)。 但是,有一个简单的转换可以消除这个限制。假设工作人员比任务少,我们添加一些虚拟人员(足以使生成的图形成为完整的二分图)。每个虚拟工人都有从工作人员到每个任务的弧。每个这样的弧都有重量0(放在匹配中,没有增加利润)。在这个变化之后,图形是一个完整的二分图,我们可以解决。任何分配了虚拟工作者的任务都不会启动。 假设有更多的工作比工人。我们添加一些虚拟任务(足以使生成的图形成为完整的二分图)。每个虚拟任务都具有从每个工作人员到虚拟任务的弧。每个这样的弧度的权重为0(将其放置在匹配中不产生附加利润)。在这个变化之后,图形是一个完整的二分图,我们可以解决。在此期间,任何分配给虚拟任务的工作人员都不被雇用。
线性分配示例
最后,让我们用一直使用的代码来做一个例子。我将从这里修改示例问题。我们有3个任务:我们需要清洁浴室,扫地板,洗窗户。 可以使用的工人有爱丽丝,鲍勃,查理和黛安娜。每个工人给我们每个任务所需的工资。这是每个工人的工资:
| | 清洁浴室 | 扫地 | 擦窗户 |
| --- | ---- | --- | --- |
| 爱丽丝 | $ 2 | $ 3 | $ 3 |
| 短发 | $ 3 | $ 2 | $ 3 |
| 查理 | $ 3 | $ 3 | $ 2 |
| 黛安 | $ 9 | $ 9 | $ 1 |
如果我们要支付最少的钱,但仍然完成所有的工作,谁应该做什么任务?首先介绍一个虚拟任务,使图表代表问题二分。
| | 清洁浴室 | 扫地 | 擦窗户 | 没做什么 |
| --- | ---- | --- | --- | ---- |
| 爱丽丝 | $ 2 | $ 3 | $ 3 | $ 0 |
| 短发 | $ 3 | $ 2 | $ 3 | $ 0 |
| 查理 | $ 3 | $ 3 | $ 2 | $ 0 |
| 黛安 | $ 9 | $ 9 | $ 1 | $ 0 |
假设问题以有向图编码,kuhn_munkres( bipartition(G) )
则会解决问题并返回分配。很容易验证最优(最低成本)的分配费用为5美元。 以下是上面代码生成的解决方案的可视化: 这就对了。你现在知道你需要知道的关于线性分配问题的一切。 您可以在GitHub上找到本文中的所有代码。
参考资料
对于数据结构中的图了解不多,导致有些东西不太清楚,顺便将一些搜到参考资料记录下。另外,到后期暂时实在看不下去了,所以强烈推荐各位最好去啃原文。
除特别注明外,本站所有文章均为 windcoder 原创,转载请注明出处来自: zuidaliulianghexianxingfenpeiwenti
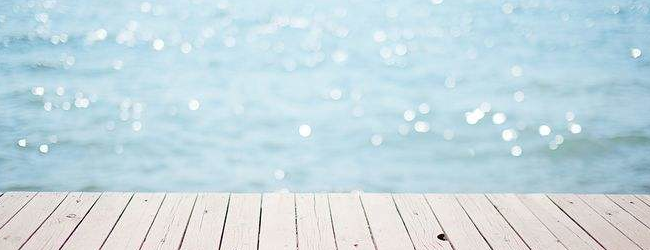
暂无数据